Electron Tomography Segmentation
Cryo-electron tomography (cryo-ET) is a powerful imaging technique used to study the three-dimensional (3D) cellular components and macromolecular complexes in their near-native state, providing insights into their organization and interactions. The data acquired can be noisy, and the sample may undergo distortions during the imaging process.
Using Machine Learning models like U-Net, Segment Anything (SAM) and Autoencoder, we are able to successly segment the cellular structures like ribosome, membrane, microtubules, filament.
My Contributions:
- Designed end-to-end pipeline for semantic segmentation of low resolution and sparse cellular structures from cyro electron tomography images using Multi-UNet architecture, resulting in a significant 13% boost in F1-score
- Developed a 2-stage semi-supervised segmentation framework to segment cellular regions and fine-grained cellular structures, reducing the number of manual annotations by 85%
- Developed unsupervised algorithms to effectively segment different instances of chromosomes in a nucleus
- Designed advanced image processing pipelines for precise 3D pose extraction of cellualr structures from volumetric microscopic imaging data, leveraging connected components, spline interpolation, and geometric transformations
- Integrated fine-tuned Segment Anything Model (SAM) in the pipeline, eliminating manual parameter tuning and enhancing the performance of the segmentation masks by 20%
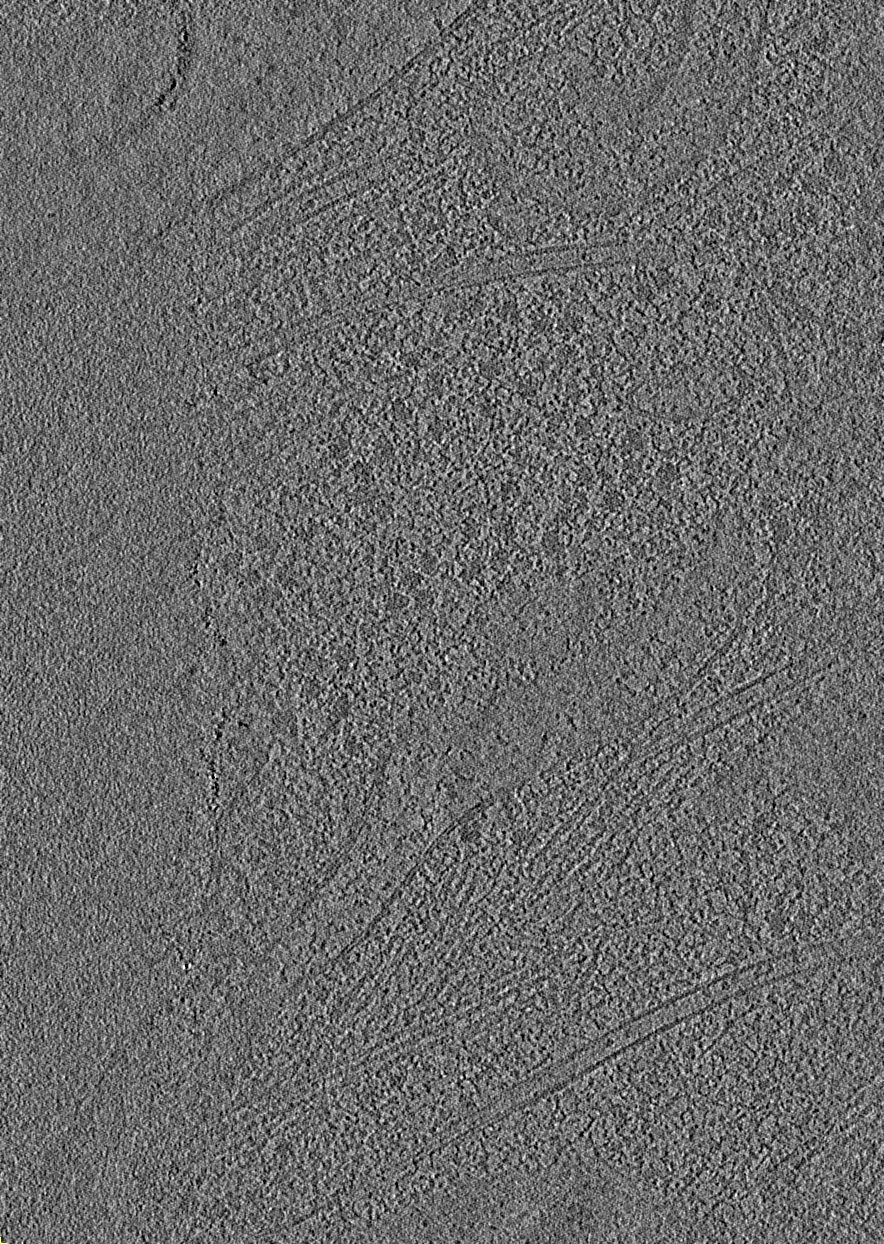
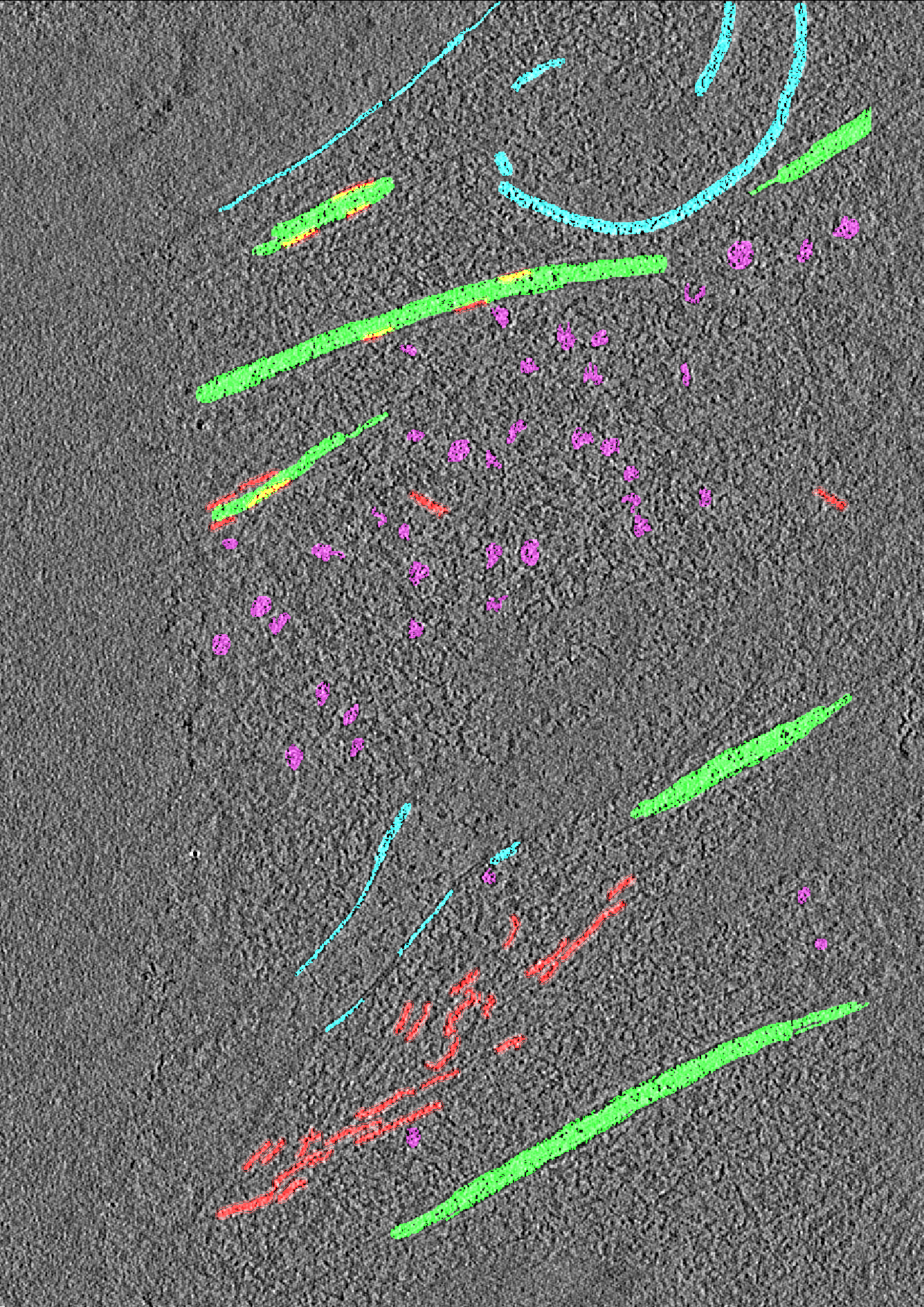
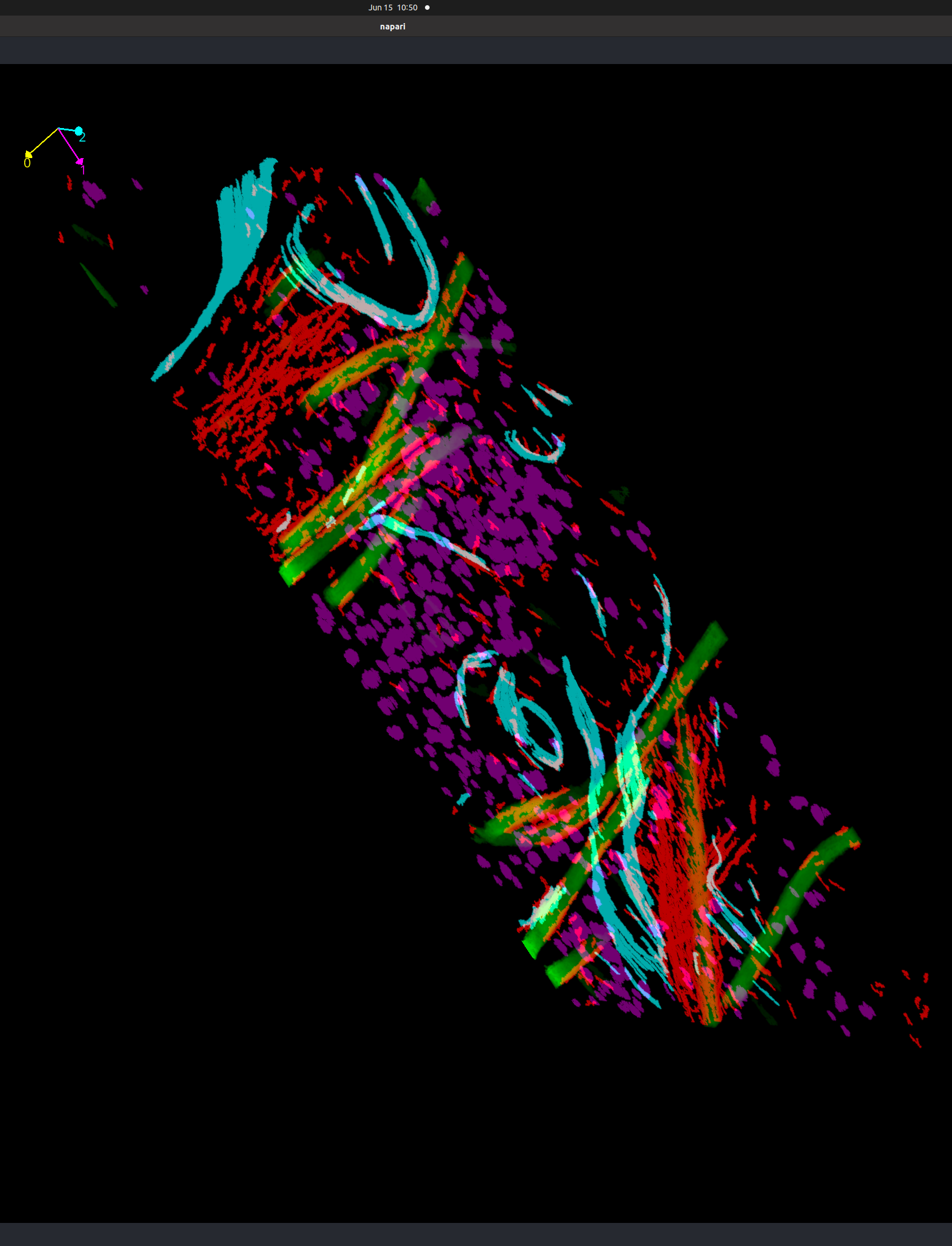